Similarities Between Human Brain Neurons and Artificial Neural Networks
- Caner Çetin
- Aug 18, 2024
- 5 min read
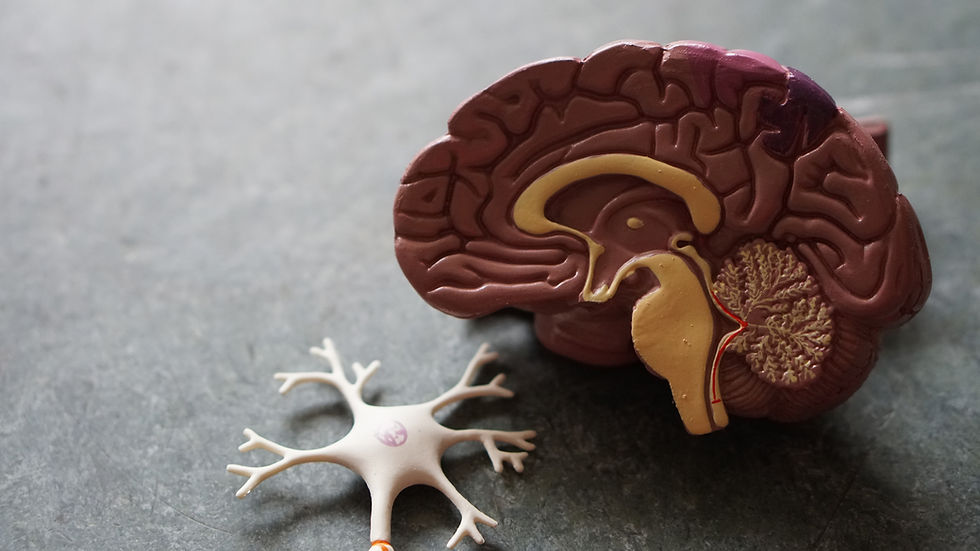
Our human brain comprises a lot of neuron cells, and these cells make connections with each other. This process means that we have the plasticity to adapt to new life conditions. We have different types of nervous systems, such as the central nervous system and the peripheral nervous system. Each of them has special features.
I. Biological Human Brain Neurons
Our general nervous system comprises two different systems: the central nervous system (CNS), and the peripheral nervous system (PNS). The first of them has a special function in the body. The CNS includes the brain and spinal cord, while the peripheral system includes other areas. Our unique brains have a lot of parts that have diverse functions and anatomy. Especially, the cerebellum has a crucial role in sending messages to the body through the spinal cord; therefore, the cerebellum is responsible for communication between the frontal motor cortex and messages that come from the peripheral nervous system. It has a mission to direct arm and leg coordination (Thau et al., 2022). The second of them is structure, which includes peripheral nerves, ganglia, and sensory receptors. The PNS contains 12 pairs of cranial nerves that make connections between particular body parts and the CNS (Paz & West, 2014: 129).
Human brain neurons are specialized cells to transmit knowledge and impulses between two different neuron cells. Neurons need someparticular parts to communicate witheach other. Firstly, some are necessary to store information of neurons and it is responsible for protecting and evaluating information that arrived or went . Taking message that came from other neurons, neurons have too many dendrites. These dendrites can be found ready to get information from other neurons. Indeed, every neuron has only one axon to send message to another neuron. It is always longer than dendrites. All of the neurons have personal mission in one complex and entire brain (Bazira, 2021: 451).
Human brain neurons work when they are activated to start communication among neuron cells. It means they need to activate, and this is called activation potential. To activate activation potential, neurons need some requirements such as amount of potassium and sodium. Generally, neuron cells have high concentration sodium ion inside of cell and have high concentration potassium levels outside of neuron (Larkman & Barnett, 2007: 192).
When the activation potential is activated through three sodium ions inside of the neuron exchanged for two potassium ions the outside, synaptic connection is completed, and information is passed to postsynaptic as chemical structure. Also, each of the channels are selective permeable and it means every channel has just one function like one channel only permits the flow of sodium while other channel only permits the flow of potassium. In this context, knowing that a sodium ion is about 30 percent smaller than the potassium (Stevens, 1979: 55-58) and the resting value of neuron is -70 millivolts. Then, if neuron membrane increasesand activates activation potential, that is depolarization; if neuron membrane decreasesand drops below of resting membrane,that is hyperpolarization (Larkman & Barnett, 2007: 193).
II. Artificial Neural Networks
Artificial Neural Networks (ANNs) are an imitation of biological neuron cells. They have similar models to process input that come from outside. ANNs also have a learning style like the brain, and this works with errors and learns from mistakes that happen in the process. After detecting the difference between input and expected output, ANNs are adjusting their own connections (Dongare et al., 2012: 190). It is a generalized running discipline. So, we need to talk about learning types and diversity among each other.
In literature; supervised, unsupervised, and reinforced learning types become prominent. According to Dongare et al. (2012: 190), supervised learning means a system has a sample to classify input and to adapt to expected output. In this process, the algorithm tries to find which one is correct or similar to the expected output. In unsupervised learning, the algorithm does not have an expected output, so it tries to classify similarities on by means of input information. It tries to realize diversity and similarity of input and try to emerge a classification method. We can use the word grouping instead of classifying. Lastly, reinforced learning works like conditional learning because this style needs to get feedback from the environment. It works with reward or punish principles; therefore, reinforced learning is based on environmental response, and it adjusts the system to its parameters.
Supervised learning includes things like learning the name of a fruit or which one is yellow with the help of a teacher. In there, algorithms have a mentor and an expected output. The system keeps identifying and figuring out which one goes with yellow or what kind of name it has. In unsupervised learning, algorithms do not have an expected answer. They just try to detect similarities between different pictures. If you provide data with some pictures that include cat and dog pictures, the unsupervised learning model will try to detect the differences, and which one is a cat or a dog. In reinforced learning, algorithms run to get higher points. So, it is similar to gaining some achievements in a game. Reinforced algorithms try each condition, and they begin to learn which event is improving scores and which one is decreasing scores.
According to the studies of Wu and Feng (2017: 1650-1653), when we look to applications and using areas of ANNs, we can see too many using areas:
Information Processing: ANNs have high fault tolerance. They have a function to classify, and we see them in automatic tracking and monitoring instrumentation systems, automatic control guidance systems, and alarm systems.
Pattern Recognition: Artificial neural networks pattern cognition replaces the traditional pattern cognition method. It has a lot of uses in areas such as character recognition, speech recognition, face cognition, and recognition of handwritten characters.
Biological Signal Detection and Analysis: ANNs are flexible to adapt to new situations to detect differences and deficits. Specially in this area, ANNs focus on the analysis of EEG signals, compression of ECK signals, and finally recognition of medical images.
Risk Assessment: In commerce, earning profit is a purpose, and predicting what will happen in the future is a method to keep growing. So, ANNs are open to seeing sales records and predicting what is necessary to earn more money.
Transport Applications: There are some important factors about transport, such as vehicle type, driver, and pavement. So, projects focus on vehicle driver behaviour simulation, pavement maintenance, vehicle detection and classification, economy, and transportation strategy.
Psychology: ANNs depend on functions of neurons and imitate memory, cognition, learning, and other cognitive processes. ANNs use to understand the brain's memory, learning, and all functions by creating process mechanisms.
References
A. D. Dongare, R. R. Kharde, & D. Kachare, A. (2012). Introduction to Artificial Neural Network. International Journal of Engineering and Innovative Technology 2(1), 188-194.
Barnett, MV. & Larkman, PM. (2007). The Action Potential. Practical Neurology 7(3), 192-197.
Bazira, P. J. (2021). An Overview of the Nervous System. Surgery, 39(8), 451–462. https://doi.org/10.1016/j.mpsur.2021.06.012
Paz, J. C. & West M. P. (2019). Acute Care Handbook for Physical Therapists. Elsevier.
Stevens, C. F. (1979). The Neuron. Scientific American, 241(3), 54–65. http://www.jstor.org/stable/24965287
Thau, L., Reddy, V. & Singh P. (2022). Anatomy, Central Nervous System. Stat Pearls.
Wu, Y. C., & Feng, J. W. (2017). Development and Application of Artificial Neural Network. Wireless Personal Communications, 102(2), 1645–1656. https://doi.org/10.1007/s11277-017-5224-x
Comments